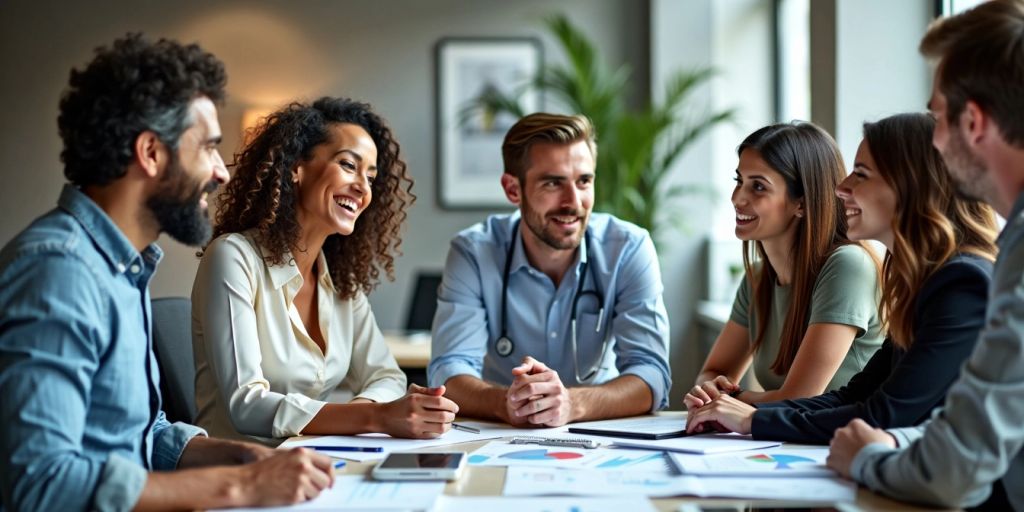
Understanding Price Forecasting: Techniques and Best Practises for Accurate Predictions
Price forecasting is a vital tool that helps businesses predict future price movements based on past data and market trends. Understanding the methods and best practises in this field can significantly enhance decision-making processes, enabling companies to optimise their pricing strategies and maintain a competitive edge. This article explores various forecasting techniques, technological advancements, and practical applications across different industries.
Key Takeaways
- Price forecasting is crucial for making informed business decisions.
- Different methods, including statistical and machine learning techniques, offer unique advantages.
- Regular updates and collaboration across departments improve forecasting accuracy.
- Understanding market trends and consumer behaviour is essential for effective price predictions.
- Ethical considerations and data quality are critical for reliable forecasting outcomes.
What is Price Forecasting?
Definition and Importance
Price forecasting is all about predicting future price movements of goods or services. We base these predictions on historical data, market trends, and various external factors. This process is crucial for making informed decisions regarding pricing, inventory management, and budget planning. By understanding market dynamics, we can optimise our pricing strategies and improve profit margins.
Key Objectives
The main goals of price forecasting include:
- Enhancing decision-making: Helps businesses make informed choices.
- Optimising pricing strategies: Ensures competitive pricing in the market.
- Improving profit margins: Aids in maximising revenue through better pricing.
Applications in Various Industries
Price forecasting finds its place in numerous sectors, such as:
- Retail: Helps in setting prices for products based on demand.
- Real Estate: Assists in predicting property values over time.
- Travel and Hospitality: Guides pricing for flights and hotel bookings.
Price forecasting is not just about numbers; it’s about understanding the market and making informed choices that can lead to success.
In summary, price forecasting is a vital tool that helps us navigate the complexities of market dynamics and make strategic decisions that benefit our businesses.
Approaches to Price Forecasting
When we dive into the world of price forecasting, we discover various methods that help us predict future prices. Each approach has its strengths and weaknesses, and understanding these can significantly enhance our forecasting accuracy.
Statistical Techniques
Statistical techniques are the traditional methods we often rely on. They use historical data to make predictions about future prices. Here are some common statistical methods:
- Time Series Analysis: This method looks at data over time to identify trends and patterns. It’s particularly useful for short-term forecasts.
- Regression Analysis: This technique examines the relationship between price and other influencing factors, such as supply and demand.
- Econometric Models: These models combine economic theory with statistical methods to forecast prices based on various economic indicators.
Machine Learning Techniques
On the other hand, machine learning techniques represent a more modern approach. They can handle large datasets and identify complex patterns that traditional methods might miss. Some popular machine learning methods include:
- Neural Networks: These algorithms mimic the human brain to learn from data and improve predictions over time.
- Decision Trees: This method breaks down data into branches to make decisions based on various conditions.
- Ensemble Methods: These combine multiple models to improve accuracy and robustness.
Comparative Analysis of Methods
To choose the best approach, we should consider the context and data available. Here’s a quick comparison:
Method Type | Strengths | Weaknesses |
---|---|---|
Statistical | Simple, interpretable | May not capture complex patterns |
Machine Learning | Handles large datasets, identifies patterns | Requires more data and computational power |
In conclusion, the choice of method depends on the specific needs of our forecasting task. By understanding both statistical and machine learning techniques, we can better navigate the complexities of price forecasting and make informed decisions.
Steps in Implementing Price Forecasting
When we dive into price forecasting, we need to follow a clear set of steps to ensure our predictions are spot on. Here’s how we can do it:
Data Collection and Preparation
First off, we need to gather comprehensive data. This includes:
- Historical price data
- Market trends
- Economic indicators
- Consumer behaviour insights
The more detailed our data, the better our analysis will be.
Choosing the Right Model
Next, we have to pick a forecasting model that fits our data. This could be:
- Statistical models like regression analysis for simpler datasets
- Machine learning models for more complex data
Choosing the right model is crucial for accurate predictions.
Model Training and Testing
Once we have our model, it’s time to train it. We’ll feed our data into the model and let it learn to spot patterns. We should split our data into:
- Training set
- Testing set
This helps us check how well our model can predict future prices.
Forecasting and Analysis
After training, we can use the model to forecast future prices. It’s important to analyse these forecasts in light of current market conditions. This way, we can make informed decisions about pricing strategies.
Continuous Monitoring and Adjustment
Finally, we must remember that price forecasting isn’t a one-off task. We need to regularly monitor our model’s performance and adjust it as new data comes in. This ensures our forecasts remain relevant and accurate.
By following these steps, we can enhance our forecasting accuracy and make better business decisions. Understanding the process is key to success!
Best Practises for Accurate Price Forecasting
Understanding Market Dynamics
To make our price forecasting more effective, we need to understand market dynamics. This means looking at how external factors like economic changes and competitor actions can affect prices. For instance, if a new technology trend emerges, we should consider how it might influence our pricing strategies. By keeping an eye on these dynamics, we can better predict price points that will appeal to our customers and help us stay competitive.
Diversifying Data Sources
We should also diversify our data sources. Relying on a single type of data can limit our understanding. Instead, we can combine various sources such as:
- Internal sales data
- Market research
- Industry reports
This mix gives us a fuller picture, helping us forecast prices that align with what consumers want and current market trends.
Regular Model Updates
Keeping our forecasting model updated is crucial. We need to ensure it reflects the latest data and market insights. For example, an airline might regularly adjust its pricing model based on current fuel costs and flight demand. This practise helps maintain the accuracy of our forecasts.
Cross-Functional Collaboration
Finally, we should encourage cross-functional collaboration. Involving different departments can provide valuable insights. For example, in a software company, the sales, marketing, and product teams can work together to understand how new features might affect pricing. This teamwork can lead to more accurate pricing strategies.
By following these best practises, we can enhance our price forecasting efforts and make more informed decisions that benefit our business.
Technological Advancements in Price Forecasting
Role of AI and Machine Learning
In recent years, AI and machine learning have transformed how we approach price forecasting. These technologies allow us to analyse vast amounts of data quickly and accurately. They can identify complex patterns that traditional methods might miss. For instance, data-driven stock forecasting models based on neural networks have shown remarkable results in predicting price movements. This means we can make better decisions based on more reliable forecasts.
Tools and Software
There are numerous tools and software available that enhance our forecasting capabilities. Here are some popular types:
- Time Series Analysis Tools: These help us track changes over time, identifying trends and seasonal patterns.
- Predictive Analytics Software: These use algorithms to forecast future prices based on historical data.
- Visualisation Tools: These turn complex data into easy-to-understand insights, making it simpler for us to make informed decisions.
Tool Type | Purpose |
---|---|
Time Series Analysis | Identifies trends over time |
Predictive Analytics | Forecasts future prices |
Visualisation Tools | Simplifies complex data for decision-making |
Integration of Big Data
The integration of big data into price forecasting is crucial. By combining various data sources, we can gain a more comprehensive view of market dynamics. This includes:
- Historical sales data
- Market research reports
- Social media trends
By leveraging big data, we can enhance our forecasting accuracy and adapt to market changes more effectively.
In summary, the advancements in technology, particularly in AI and big data, are reshaping how we forecast prices. By embracing these tools and techniques, we can improve our predictions and make more informed business decisions.
Challenges and Ethical Considerations
Data Quality Issues
When we think about price forecasting, one of the biggest hurdles we face is data quality. Poor data can lead to inaccurate predictions, which can be costly. Here are some common data quality issues:
- Incomplete data sets
- Outdated information
- Inconsistent data formats
Human Factors
Another challenge is the human factor. People can introduce bias into forecasting models, whether intentionally or not. This can skew results and lead to poor decision-making. We need to be aware of:
- Personal biases in data interpretation
- Miscommunication between teams
- Over-reliance on historical data without considering current trends
Ethical Use of Data
Finally, we must consider the ethical use of data. As we gather and analyse data, we must ensure that we are not infringing on privacy rights or using data in a way that could harm individuals or groups. This includes:
- Ensuring transparency in data usage
- Avoiding discrimination in pricing strategies
- Complying with regulations like GDPR
In our pursuit of accurate price forecasting, we must remember that ethical considerations are just as important as technical skills.
By addressing these challenges, we can improve our forecasting accuracy and maintain trust with our stakeholders. Ethical considerations are crucial in ensuring that our models are fair and unbiased, ultimately leading to better outcomes for everyone involved.
Case Studies and Real-World Applications
Retail Industry
In the retail sector, price forecasting plays a crucial role in inventory management and sales strategies. By analysing historical sales data, retailers can predict future demand and adjust their pricing accordingly. For instance, many retailers use seasonal trends to forecast sales during holidays, ensuring they stock the right amount of products. This helps in minimising excess inventory and maximising sales potential.
Real Estate Market
The real estate market also benefits significantly from price forecasting. By employing econometric models, analysts can predict property values based on various factors such as location, economic conditions, and interest rates. This allows investors to make informed decisions. For example, predicting energy prices based on a novel hybrid machine learning model can help stakeholders understand potential future costs associated with properties, influencing buying and selling strategies.
Travel and Hospitality Sector
In the travel and hospitality industry, price forecasting is essential for setting competitive rates. Companies analyse historical booking data and market trends to predict future demand. This enables them to adjust prices dynamically, ensuring they remain attractive to customers while maximising revenue. For instance, airlines often use sophisticated algorithms to forecast ticket prices based on factors like seasonality and competitor pricing.
Industry | Key Techniques Used | Benefits |
---|---|---|
Retail | Historical sales analysis | Optimised inventory management |
Real Estate | Econometric models | Informed investment decisions |
Travel and Hospitality | Dynamic pricing algorithms | Maximised revenue through competitive rates |
In our experience, understanding the specific needs of each industry is vital for effective price forecasting. By tailoring our approaches, we can achieve more accurate predictions and better outcomes for our clients.
In our section on case studies, we showcase how businesses like yours have successfully implemented AI solutions. These real-world examples highlight the benefits and transformations that AI can bring. If you’re curious about how AI can work for you, visit our website to learn more and start your journey today!
Conclusion
In summary, price forecasting is a valuable tool for both businesses and consumers. It helps in making smart choices for daily operations and long-term plans. By using price forecasting tools, users are encouraged to interact with brands and assess offers, ensuring they spend their money wisely.
To be effective in price forecasting, analysts need to understand the factors that influence demand for products and services. These factors can include seasonal changes, holidays, daily activities, political and economic conditions, and even weather patterns. Additionally, it is crucial to be aware of the marketing strategies and pricing techniques used by competitors.
Another key to success is having access to high-quality, up-to-date data. Analysts must gather enough information to build and test predictive models, as well as maintain a solid data management strategy. Therefore, the choice of methods and techniques will depend on the type of data available.
Frequently Asked Questions
What does price forecasting mean?
Price forecasting means trying to guess what future prices of products or services will be based on past data and market trends.
Why is price forecasting important?
It helps businesses make smart choices about pricing, managing stock, and planning budgets.
What methods are used for price forecasting?
There are different ways to forecast prices, such as using statistical methods or machine learning techniques.
How can companies improve their price forecasts?
By understanding market trends, using various data sources, updating models regularly, and working with different teams.
What challenges do businesses face in price forecasting?
Some challenges include data quality issues, unpredictable human behaviour, and ethical concerns regarding data use.
Can you give examples of industries that use price forecasting?
Yes, industries like retail, real estate, and travel often use price forecasting to make informed decisions.