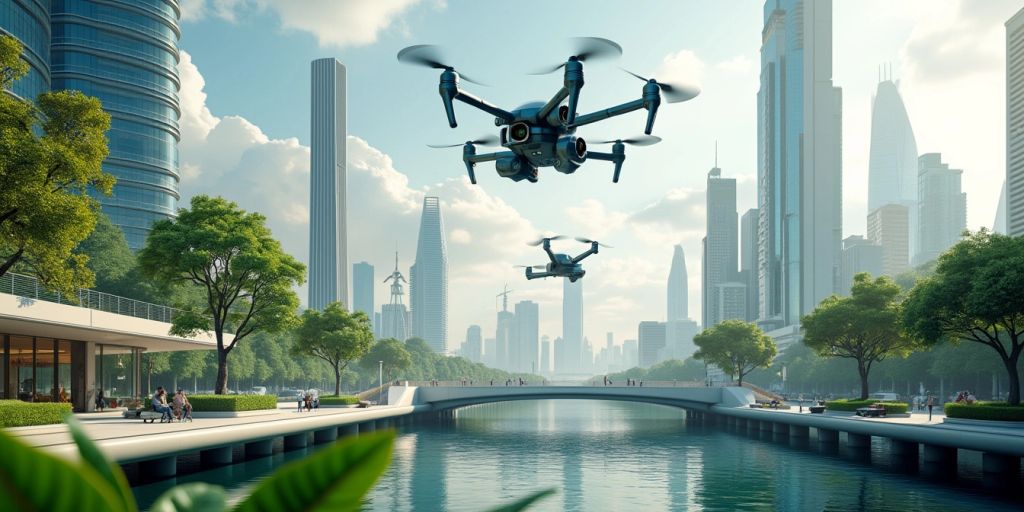
Navigating the Future: A Guide to Risk-Free AI Implementation
As organisations increasingly integrate Artificial Intelligence (AI) into their operations, understanding how to implement it safely and ethically is crucial. This guide aims to provide insights into achieving risk-free AI implementation, focusing on essential strategies and best practises. By navigating the complexities of AI with care, businesses can harness its potential while safeguarding their interests and those of their stakeholders.
Key Takeaways
- AI implementation should prioritise ethical practises to foster trust.
- Organisations must assess their readiness for AI adoption before integration.
- Data privacy and security are critical in AI deployment strategies.
- Transparency in AI processes helps build stakeholder confidence.
- Continuous monitoring and adaptive measures are essential for sustainable AI practises.
Understanding the Foundations of Risk-Free AI Implementation
Defining Risk-Free AI
When we talk about risk-free AI, we mean implementing AI systems that minimise potential dangers while maximising benefits. This involves understanding the risks associated with AI and taking steps to mitigate them. Here are some key points to consider:
- Clear objectives: Define what we want AI to achieve.
- Thorough assessments: Regularly evaluate risks and benefits.
- Stakeholder involvement: Engage all relevant parties in the process.
Importance of Ethical Considerations
Ethics play a crucial role in AI implementation. We must ensure that our AI systems are not only effective but also fair and just. Here are some ethical considerations:
- Bias minimisation: Actively work to reduce bias in AI algorithms.
- Transparency: Ensure that AI decision-making processes are clear.
- Accountability: Establish who is responsible for AI outcomes.
Key Components of AI Governance
To navigate the complexities of AI, we need a solid governance framework. This includes:
- Policies and guidelines: Develop clear rules for AI use.
- Risk management strategies: Implement robust strategies for identifying and managing risks, as highlighted in our reference to risk management strategies for AI implementation.
- Continuous improvement: Regularly update our governance practises based on new insights and technologies.
In summary, understanding the foundations of risk-free AI implementation is essential for harnessing its full potential while safeguarding against its inherent risks. By focusing on clear definitions, ethical considerations, and strong governance, we can create a safer AI landscape for everyone.
Strategic Planning for AI Integration
Assessing Organisational Readiness
Before diving into AI integration, we need to evaluate our organisational readiness. This involves:
- Understanding our current technological capabilities.
- Identifying the skills and knowledge gaps within our team.
- Assessing our existing data infrastructure to support AI initiatives.
Developing a Comprehensive AI Strategy
Creating a solid AI strategy is crucial for success. Here’s how we can approach it:
- Define clear objectives that align with our business goals.
- Identify the specific AI technologies that can drive value.
- Establish a timeline and budget for implementation.
Aligning AI Initiatives with Business Goals
To ensure our AI projects are effective, we must:
- Ensure that every AI initiative directly supports our core business objectives.
- Regularly review and adjust our strategies based on performance metrics.
- Foster collaboration between departments to enhance AI integration.
By taking these steps, we can create a robust framework for AI integration that not only meets our needs but also positions us for future success.
In summary, strategic planning is essential for a smooth AI integration process. We must assess our readiness, develop a comprehensive strategy, and align our initiatives with our business goals to navigate this exciting journey successfully.
Ensuring Data Privacy and Security
In our journey towards effective AI implementation, data privacy and security are non-negotiable. We must ensure that the data we use is not only secure but also respects the privacy of individuals. Here’s how we can achieve this:
Implementing Robust Data Governance
To safeguard our data, we need to establish strong data governance policies. This includes:
- Setting clear guidelines for data collection and storage.
- Ensuring compliance with privacy laws.
- Using encryption to protect sensitive information.
By doing this, we not only enhance the quality of our AI models but also build trust with our users.
Addressing Data Privacy Concerns
As we explore AI’s impact on data privacy, we must be aware of the growing data privacy concerns. Here are some steps we can take:
- Regularly review our data handling practises.
- Educate our teams about data privacy regulations.
- Implement measures to anonymise personal data.
These actions will help us mitigate risks and protect personal information in an AI-driven world.
Mitigating Security Risks
To ensure our AI systems are secure, we should:
- Conduct regular security audits.
- Stay updated on the latest cybersecurity threats.
- Foster a culture of security awareness within our teams.
By prioritising these practises, we can significantly reduce the chances of data breaches and maintain the integrity of our AI systems.
In the end, our commitment to data privacy and security will not only protect our users but also enhance the credibility of our AI initiatives.
By focusing on these areas, we can navigate the complexities of AI implementation while ensuring that we respect and protect the data of individuals.
Enhancing Transparency and Accountability
Promoting Explainable AI
In our journey towards effective AI implementation, promoting explainable AI is crucial. We need to ensure that everyone involved understands how AI systems make decisions. This can be achieved by:
- Creating user-friendly interfaces that clarify AI recommendations.
- Publishing clear documentation on AI models and their functionalities.
- Hosting open discussions to share insights and outcomes of AI strategies.
Establishing Clear Accountability Frameworks
To build trust, we must establish clear accountability frameworks. This means defining who is responsible for AI decisions and outcomes. Here are some steps we can take:
- Identify key stakeholders involved in AI governance.
- Develop guidelines that outline roles and responsibilities.
- Ensure regular reviews of AI systems to maintain accountability.
Fostering Stakeholder Trust
Fostering trust among stakeholders is essential for successful AI integration. We can achieve this by:
- Engaging diverse groups in the AI governance process.
- Addressing concerns and feedback from users and affected communities.
- Ensuring transparency in how data is used and decisions are made.
By focusing on transparency and accountability, we can navigate the complexities of AI and ensure that our systems are not only effective but also ethical and trustworthy.
In summary, enhancing transparency and accountability in AI systems is not just about compliance; it’s about building a foundation of trust that will support the sustainable growth of AI technologies. This trust is vital for the future of AI in our society.
Overcoming Challenges in AI Deployment
When we think about overcoming challenges in AI deployment, it’s clear that we face a few hurdles. Here’s how we can tackle them:
Navigating Regulatory Complexities
- Understand the laws: Each country has its own rules about AI. We need to know what they are to avoid problems.
- Stay updated: Laws change often, so we must keep an eye on new regulations.
- Engage with regulators: Building relationships with those who make the rules can help us stay ahead.
Managing Technological Integration
- Assess current systems: Before adding AI, we should check if our existing technology can support it.
- Plan for training: Our team needs to learn how to use new AI tools effectively.
- Test before full rollout: Starting with a small pilot can help us identify issues early.
Addressing Ethical and Social Implications
- Promote fairness: We must ensure our AI systems treat everyone equally and do not reinforce biases.
- Involve diverse voices: Getting input from various stakeholders can help us understand different perspectives.
- Be transparent: Sharing how our AI systems work builds trust with users.
By recognising and tackling these challenges, we can create a smoother path for AI deployment. Our organisation must be certain they can achieve this adoption to avoid delays or setbacks.
In summary, overcoming challenges in AI deployment requires a proactive approach, continuous learning, and collaboration across all levels of our organisation. Let’s work together to make AI a beneficial tool for everyone!
Future Trends in AI Risk Management
As we look ahead, the landscape of AI in risk management is evolving rapidly. The integration of AI in risk management will continue to grow, with researchers examining even more advanced applications such as explainable AI to boost transparency and trust.
Advancements in Machine Learning
- We can expect to see more sophisticated machine learning techniques.
- Deep learning and reinforcement learning will enhance predictive capabilities.
- These advancements will allow for more accurate risk assessments, helping us make better decisions.
Integration of Quantum Computing
- Quantum computing is on the rise, promising to solve complex problems.
- Its integration with AI could significantly speed up risk assessments.
- This technology will help us tackle challenges that traditional computing struggles with.
Emerging Predictive Analytics Techniques
- New predictive analytics methods will emerge, improving our ability to foresee risks.
- These techniques will leverage vast amounts of data for better insights.
- We will be able to implement preventive measures more effectively.
In this ever-changing environment, staying ahead of trends is crucial for effective risk management. By embracing these advancements, we can navigate uncertainties with greater confidence and agility.
Best Practises for Sustainable AI Implementation
Continuous Monitoring and Evaluation
To ensure our AI systems remain effective, we must engage in continuous monitoring. This means regularly checking how our AI models perform and making necessary adjustments. Here are some steps we can take:
- Set up real-time performance tracking.
- Conduct regular audits to spot any biases or errors.
- Update models promptly to fix any identified issues.
Adaptive Security Measures
Security is crucial in AI. We need to implement adaptive security measures to protect our data and systems. This includes:
- Regularly updating security protocols.
- Training staff on the latest security practises.
- Using encryption to safeguard sensitive information.
Stakeholder Collaboration
Engaging with various stakeholders is essential for successful AI implementation. We should:
- Involve internal teams like developers and business units.
- Seek feedback from external parties such as customers and advocacy groups.
- Create open forums for discussion to ensure everyone’s voice is heard.
By recognising and addressing these challenges, we can develop more energy-efficient algorithms and optimise AI infrastructure, significantly reducing the environmental impact of AI systems.
In conclusion, by following these best practises, we can ensure that our AI initiatives are not only effective but also sustainable and responsible. Let’s work together to make AI a force for good!
To successfully implement sustainable AI, it’s essential to follow best practices that ensure efficiency and responsibility. Start by assessing your current systems and identifying areas where AI can make a positive impact. Collaborate with experts to tailor solutions that fit your needs. For more insights and to kickstart your AI journey, visit our website today!
Conclusion
In summary, using AI in risk management marks a significant change in how businesses deal with uncertainties. With tools like real-time risk assessment and predictive analytics, AI helps companies make better choices and become stronger against future challenges. Although there are hurdles to overcome, such as data privacy and the need for clear explanations of AI decisions, the ongoing progress in AI technology and a focus on responsible practises suggest a future where risk management is not just more effective but also more flexible. By embracing AI, organisations can lead the way in innovation, confidently facing the future.
Frequently Asked Questions
What does risk-free AI mean?
Risk-free AI refers to the safe use of artificial intelligence that avoids potential dangers or negative impacts on individuals or society.
Why is ethics important in AI?
Ethics in AI ensures that technology is used responsibly, promoting fairness and preventing harm to people.
What are the main parts of AI governance?
AI governance includes rules and practises that guide how AI is developed and used, focusing on safety, fairness, and accountability.
How can organisations prepare for AI?
Organisations can prepare for AI by assessing their current capabilities, creating a clear strategy, and aligning AI projects with their goals.
What should I know about data privacy with AI?
Data privacy in AI involves protecting personal information and ensuring that data is used responsibly to prevent misuse.
What is the future of AI risk management?
The future of AI risk management includes advancements in technology that improve safety and effectiveness, making AI even more reliable.